こんにちわ。
TEDの動画より医療英会話の学習に有用なスピーチを紹介します。
今回のテーマは「数学を用いた癌の治療開発」に関する内容です。
私自身も応用数学を用いた癌の研究に携わっており、非常に興味深い内容でしたのでこのテーマをピックアップさせて頂きました。
今回紹介するイリーナ・カレバは腫瘍を標的とする新薬の開発を目的として、がんのダイナミクスを数学モデリングを行うプロジェクトに参画しています。
数学と癌の研究の関連性は中々イメージし辛い点もあるかとは思いますが、早速見ていきましょう。
TEDで医療英会話を学習【Irina Kareva|数学が解明する癌の秘密】
日英翻訳全文
I write mathematical models which, in my case, are systems of differential equations, to describe biological mechanisms, such as cell growth. Essentially, it works like this.
私は「翻訳者」です。
生物学から数学へ、またその逆の 「翻訳」をします。
私は数学的なモデルを作っていまして— 私の場合は微分方程式ですが、その方法で細胞の成長のような生物学的メカニズムを表現します。
基本的にはこの様なイメージです。
First, I identify the key elements that I believe may be driving behavior over time of a particular mechanism.
Then, I formulate assumptions about how these elements interact with each other and with their environment.
まず、あるメカニズムを動かしているものが何かその基本的要素を探し出します。
その上で、それらの要素がお互いに、また周囲とどの様に相互作用するのか、仮説を立てます。
It may look something like this.
Then, I translate these assumptions into equations, which may look something like this.
Finally, I analyze my equations and translate the results back into the language of biology.
たとえばこの様な感じです。
さらにその仮定を方程式に書き直します。
たとえばこの様な感じの最後に、その方程式を分析し 、結果を生物学の言葉へと翻訳します。
00:51
数学的モデルを作る上で重要なポイントは、物事が何であるかを考えるのではなく、その挙動を考えるということです。
We think about relationships between individuals, whether they be cells, animals or people, and how they interact with each other and with their environment.
対象が細胞であれ、動物や人間であれ、各要素の間の関係がどうなっているのか、互いや周囲に対してどの様に相互作用しているのかを考えます。
Let me give you an example. What do foxes and immune cells have in common?
They're both predators, except foxes feed on rabbits, and immune cells feed on invaders, such as cancer cells.
But from a mathematical point of view, a qualitatively same system of predator-prey type equations will describe interactions between foxes and rabbits and cancer and immune cells.
例を挙げましょう。
キツネと免疫細胞に共通するものは何でしょうか。
それはどちらも捕食者だということです。
異なる点は、キツネが食べるのはウサギで、免疫細胞が食べるのはがん細胞という点です。
しかし数学的観点からすると、質的には同じ捕食者-被捕食者の関係を表す方程式によって、キツネとウサギの関係もがんと免疫細胞の関係を表現する事が出来ます。
01:37
捕食者-被捕食者のシステムについては研究論文が多くあり、2つの集団の一方の生存が、他方を食べることに依存する場合の相互作用として記述されています。
And these same equations provide a framework for understanding cancer-immune interactions, where cancer is the prey, and the immune system is the predator.
それと同様の方程式が、がんと免疫細胞の相互作用を理解する枠組みを与えてくれて、そこではがんが被捕食者、免疫細胞が捕食者の関係になっています。
And the prey employs all sorts of tricks to prevent the predator from killing it, ranging from camouflaging itself to stealing the predator's food.
被捕食者は捕食者に殺されまいとして、あらゆることをします。
例えば自らを偽装することから、捕食者の食べ物を盗むこと等です。
This can have some very interesting implications.
For example, despite enormous successes in the field of immunotherapy, there still remains somewhat limited efficacy when it comes solid tumors.
これは様々な興味深いことを意味します。
たとえば 免疫療法は大きな成果を挙げていますが、固形腫瘍に関しては依然として、限定的な効果しか示されていません。
But if you think about it ecologically, both cancer and immune cells -- the prey and the predator -- require nutrients such as glucose to survive.
しかし生態学的に考えるのであれば、がんと免疫細胞— 被捕食者と捕食者は いずれも生存のために ブドウ糖のような栄養を必要とします 。
If cancer cells outcompete the immune cells for shared nutrients in the tumor microenvironment, then the immune cells will physically not be able to do their job.
腫瘍性の微小な環境で共有されている栄養の獲得において、もしがん細胞が免疫細胞に勝るなら、免疫細胞はその機能を果たせなくなります。
02:37
And it was recently shown experimentally that restoring the metabolic balance in the tumor microenvironment -- that is, making sure immune cells get their food -- can give them, the predators, back their edge in fighting cancer, the prey.
この「捕食者と被捕食者が共有するリソース」という形のモデルが私が研究で 取り組んできたものです。
最近 実験的に示されたことですが、腫瘍性の微小な環境の代謝のバランスを回復すると— つまり、免疫細胞がキチンと食べ物を得られるようにすると、免疫細胞は被捕食者であるがんとの戦いで優位を取り戻せるのです。
This means that if you abstract a bit, you can think about cancer itself as an ecosystem, where heterogeneous populations of cells compete and cooperate for space and nutrients, interact with predators -- the immune system -- migrate -- metastases -- all within the ecosystem of the human body.
少し抽象化して表現すると、がん組織そのものを1つの生態系と見なせる事ができ、その中で異なる種類の細胞が場所と栄養を求めて競合や協力を行い、捕食者である免疫システムと渡り合ったり、移動つまり転移をしたりしているという意味で、全ての事が人体という生態系の中で起こっているわけです。
And what do we know about most ecosystems from conservation biology? That one of the best ways to extinguish species is not to target them directly but to target their environment.
生物学の知見から、多くの生態系について言えることは何でしょうか?
それは種を絶滅させるには直接それを狙うよりも、その環境を狙うほうがより効果的だということです。
03:32
その為、腫瘍が存在する環境の主要な要素を特定できれば、仮説の作成やシナリオ、治療法のシミュレーションを安全で安価な方法で行えるようになり、微小環境の様々な要素を標的にして癌のみを殺し、私や皆さんのような宿主を傷つけない方法を探れます。
03:56
私の研究のダイレクトな目的は研究やイノベーションを進め、コストを下げることですが、本当の目的は人の命を救うことです。
And that's what I try to do through mathematical modeling applied to biology, and in particular, to the development of drugs.
私はそれを数学的モデルを生物学— 特に薬の開発に適用することで行おうとしています。
It's a field that until relatively recently has remained somewhat marginal, but it has matured. And there are now very well-developed mathematical methods, a lot of preprogrammed tools, including free ones, and an ever-increasing amount of computational power available to us.
この分野は比較的最近まであまり注目されてはいませんでしたが、今や成熟しており非常に発展した数学的手法があり、無料のものも含め沢山のソフトウェアが 作られ、使用出来る計算能力は日々増え続けています。
04:32
数学的モデルの偉力と美は、私たちが知っていると思うことを厳密な形で定式化できる点にあります。
We make assumptions, translate them into equations, run simulations, all to answer the question: In a world where my assumptions are true, what do I expect to see? It's a pretty simple conceptual framework.
私たちが仮説を作りそれを方程式に書き直し、シミュレーションを実行する時に1つの疑問が解決に向います。
自分の仮説が 正しいとしたら何を見ることになるのでしょうか?
これはすごくシンプルな概念的な枠組みです。
It's all about asking the right questions.
But it can unleash numerous opportunities for testing biological hypotheses.
まず正しい質問を問うことが 何より重要ですが、これは生物学的な仮説をテストする多くの機会をもたらしてくれます。
If our predictions match our observations, great! -- we got it right, so we can make further predictions by changing this or that aspect of the model.
もしモデルから予測されることが観察と一致するのであれば、それは素晴らしい事です。モデルがうまく機能しているということで、モデルの様々な箇所を変えて、さらに予測をすることができます。
If, however, our predictions do not match our observations, that means that some of our assumptions are wrong, and so our understanding of the key mechanisms of underlying biology is still incomplete.
しかし予測が観察と一致しないのであれば、仮定がどこか間違っていることを意味し、背後にある生物学的メカニズムの理解がどこかまだ不完全だということを意味します。
05:24
幸いこれはモデルなので、あらゆる仮定を制御できます。
So we can go through them, one by one, identifying which one or ones are causing the discrepancy.
And then we can fill this newly identified gap in knowledge using both experimental and theoretical approaches.
その為、仮定の1つひとつをチェックすると、どこで齟齬が生じているのか特定できます。
新たに見つかった 知識の穴を埋めるには、実験的手段と理論的手段の両方が使えます。
Of course, any ecosystem is extremely complex, and trying to describe all the moving parts is not only very difficult, but also not very informative.
もちろん生態系は皆非常に複雑なものですので、あらゆる変動要因を記述しようとする事は大変なだけでなく、あまり役にも立ちません。
There's also the issue of timescales, because some processes take place on a scale of seconds, some minutes, some days, months and years.
時間的尺度の問題もあります。プロセスによって数秒間のものもあれば、数分、数日、数年のものまであります。
It may not always be possible to separate those out experimentally.
And some things happen so quickly or so slowly that you may physically never be able to measure them.
実際の実験でそれを取り出すのは必ずしも可能ではありません。またあまりに早く、あるいはあまりにゆっくりと起きるため、物理的に計測することができない場合もあるかもしれません。
But as mathematicians, we have the power to zoom in on any subsystem in any timescale and simulate effects of interventions that take place in any timescale.
しかし数学者には、どんな時間尺度のどんなサブシステムにも焦点を合わせられる事が出来、どんな時間尺度で起きる効果だろうとシミュレーションをする事ができます。
06:23
Of course, this isn't the work of a modeler alone.
もちろんこれはモデル作成者が単独でやることではなく、生物学者との密な協力の元で行う必要があります。
It has to happen in close collaboration with biologists. And it does demand some capacity of translation on both sides.
But starting with a theoretical formulation of a problem can unleash numerous opportunities for testing hypotheses and simulating scenarios and therapeutic interventions, all in a completely safe way.
そしてどちらの側にも、ある程度の翻訳能力が求められます。
問題を理論的に 数式化することで仮説のテストやシナリオ、治療のシミュレーションを、非常に安全な方法で行えるという多くの可能性が開けます。
It can identify gaps in knowledge and logical inconsistencies and can help guide us as to where we should keep looking and where there may be a dead end.
どこに知識の穴や、論理的非一貫性があるかを特定する事ができ、どこに目を向けるべきか、どこが行き止まりかを 指し示してくれるのです。
06:59
別の言い方をすると、数学的モデルが人の健康に直接関わる疑問に答える助けになるということです。
個々人の健康と言った方が 良いかもしれません。
数学的モデルがオーダーメード医療を推進する鍵になるからです。
07:15
Thank you.
そしてそのためにする事は、正しい疑問点を投げかけ、適切な方程式を作り、それを生物学的に意味付けるということです。
ありがとうございました。
まとめ|今回のスピーチの内容
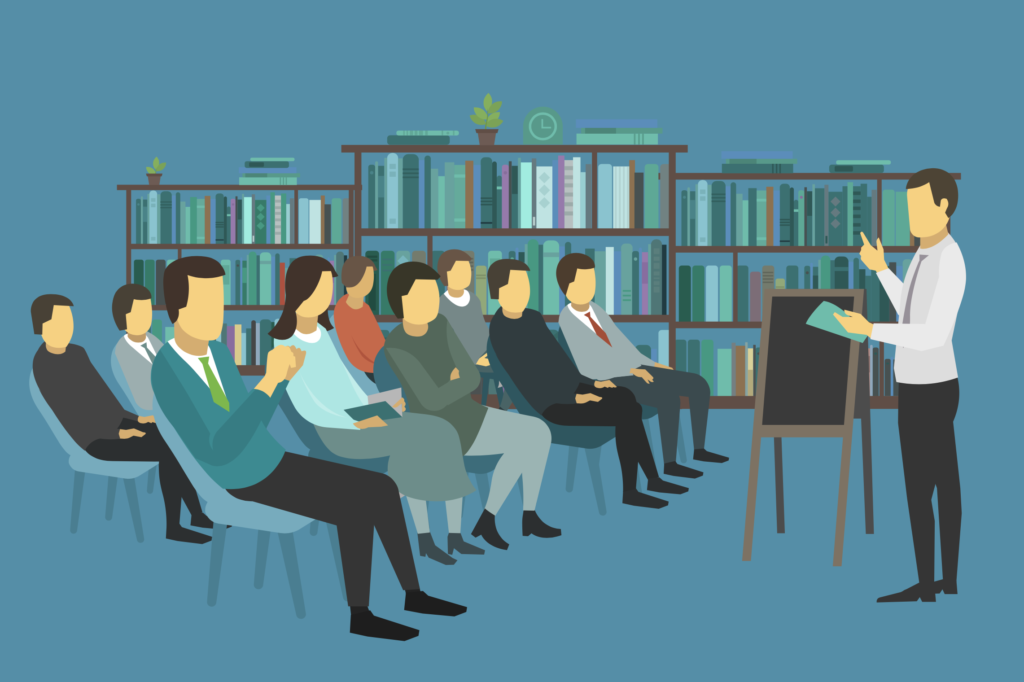
如何でしたでしょうか。
スピーチの中で、イリーナ・カレバは「癌を数学的にモデリングする表現力は、非常に厳密かつ力強くて美しく、既知の内容を数式を用いて形式化する事で、研究内容に対してどの点に着目するべきかということを明確に示してくれます。」 と述べています。
私自身も思う事ですが、数学を用いて癌の研究を行うメリットとしては、実際に臨床試験を行う上でも、患者さんに何らかの影響を与える事なく研究ができる上に、研究のコストが非常に抑えられるというメリットがあります。
具体的に使用する応用数学としては、ベイズ推定やマルコフ連鎖モンテカルロ法などがあります。日本ではこの領域に関して研究をしている方はまだ少数ですが、個人的にはAIを用いた研究だけではなく、応用数学を用いた臨床研究に将来性を感じています。
私自身も患者さんに副反応などの影響を与える事なく、シミュレーションが出来る応用数学の潜在性に魅せられて自身の研究課題にしていますが、このスピーチをきっかけに、その様な研究に興味を持って頂ければ幸いです。